RAND Corp. Finds DoD “Significantly Challenged” in AI Posture
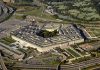
By AI Trends Staff
In a recently-released updated evaluation of the posture of the US Department of Defense (DoD) on artificial intelligence, researchers at RAND Corp. found that “despite some positive signs, the DoD’s posture is significantly challenged across all dimensions” of the assessment.
The RAND researchers were asked by Congress, within the 2019 National Defense Authorization Act (NDAA), and the director of DoD’s Joint Artificial Intelligence Center (JAIC), to help answer the question: “Is DoD ready to leverage AI technologies and take advantage of the potential associated with them, or does it need to take major steps to position itself to use those technologies effectively and safely and scale up their use?”
The term artificial intelligence was first coined in 1956 at a conference at Dartmouth College that showcased a program designed to mimic human thinking skills. Almost immediately thereafter, the Defense Advanced Research Projects Agency (DARPA) (then known as the Advanced Research Projects Agency [ARPA]), the research arm of the military, initiated several lines of research aimed at applying AI principles to defense challenges.
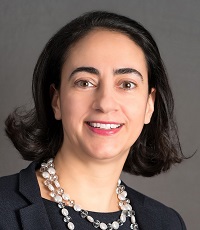
Since the 1950s, AI—and its subdiscipline of machine learning (ML)—has come to mean many different things to different people, stated the report, whose lead author is Danielle C. Tarraf, a senior information scientist at RAND and a professor at the RAND Graduate School. (RAND Corp. is a US nonprofit think tank created in 1948 to offer research and analysis to the US Armed Forces.)
For example, the 2019 NDAA cited as many as five definitions of AI. “No consensus emerged on a common definition from the dozens of interviews conducted by the RAND team for its report to Congress,” the RAND report stated.
The RAND researchers decided to remain flexible and not be bound by precise definitions. Instead, they tried to answer the question of whether the DoD is positioned to build or acquire, test, transition and sustain—at scale—a set of technologies broadly falling under the AI umbrella? And if not, what would DoD need to do to get there? Considering the implications of AI for DoD strategic decision makers, the researchers concentrated on three elements and how they interact:
- the technology and capabilities space
- the spectrum of DoD AI applications
- the investment space and time horizon.
While algorithms underpin most AI solutions, interest and hype is fueled by advances in AI, such as deep learning. This requires large data sets, and which tend to be highly-specific to the applications for which they were designed, most of which are commercial. Referring to AI verification, validation, test and evaluation (VVT&E) procedures critical to the function of software in the DoD, the researchers stated, “VVT&E remains very challenging across the board for all AI applications, including safety-critical military applications.”
The researchers divided AI applications for DoD into three groups:
- Enterprise AI, including applications such as the management of health records at military hospitals in well-controlled environments;
- Mission-Support AI, including applications such as the Algorithmic Warfare Cross-Functional Team (also known as Project Maven), which aims to use machine learning to assist humans in analyzing large volumes of imagery from video data collected in the battle theater by drones, and;
- Operational AI, including applications of AI integrated into weapon systems that must contend with dynamic, adversarial environments, and that have significant implications in the case of failure for casualties.
Realistic goals need to be set for how long AI will need to progress from demonstrations of what is possible to full-scale implementations in the field. The RAND team’s analysis suggests at-scale deployments in the:
- near term (up to five years) for enterprise AI
- middle term (five to ten years) for most mission-support AI, and
- far term (longer than ten years) for most operational AI applications.
The RAND team sees the following challenges for AI at the DoD:
- Organizationally, the current DoD AI strategy lacks both baselines and metrics for assessing progress. And the JAIC has not been given the authority, resources, and visibility needed to scale AI and its impact DoD-wide.
- Data are often lacking, and when they exist, they often lack traceability, understandability, accessibility, and interoperability.
- The current state of VVT&E for AI technologies cannot ensure the performance and safety of AI systems, especially those that are safety-critical.
- DoD lacks clear mechanisms for growing, tracking, and cultivating AI talent, a challenge that is only going to grow with the increasingly tight competition with academia, the commercial world, and other kinds of workspaces for individuals with the needed skills and training.
- Communications channels among the builders and users of AI within DoD are sparse.
The researchers made a number of recommendations to address these issues.
Two Challenge Areas Addressed
Two of these challenge areas have been recently addressed at a meeting hosted by the AFCEA, the professional association that links people in military, government, industry and academia, reported in an account in FCW. The organization engages in the “ethical exchange of information” and has roots in the US Civil War, according to its website.
Jacqueline Tame is Acting Deputy Director at the JAIC, whose years of experience include positions with the House Permanent Select Committee on Intelligence, work with an AI analytics platform for the Office of the Secretary of Defense and then positions in the JAIC. She has graduate degrees from the Naval War College and the LBJ School of Public Affairs.
She addressed how AI at DoD is running into culture and policy norms in conflict with its capability. For example, “We still have over… several thousand security classification guidance documents in the Department of Defense alone.” The result is a proliferation of “data owners.” She commented, “That is antithetical to the idea that data is a strategic asset for the department.”
She used the example of predictive maintenance, which requires analysis of data from a range of sources to be effective, as an infrastructure challenge for the DoD currently. “This is a warfighting issue,” Tame stated. “To make AI effective for warfighting applications, we have to stop thinking about it in these limited stovepiped ways.”
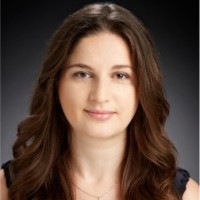
Data standards need to be set and unified, suggested speaker Jane Pinelis, the chief of testing and evaluation for the JAIC. Her background includes time at the Johns Hopkins University Applied Physics Laboratory, where she was involved in “algorithmic warfare.” She is also a veteran of the Marine Corps, where her assignments included a position in the Warfighting Lab. She holds a PhD in Statistics from the University of Michigan.
“Standards are elevated best practices and we don’t necessarily have best practices yet,” Pinelis stated. JAIC is working on it, by collecting and documenting best practices and leading a working group in the intelligence community on data collection and tagging.
Weak data readiness has been an impediment to AI for the DoD, she stated. In response, the JAIC is preparing multiple award contracts for test and evaluation and data readiness, expected soon.
Read the source articles and information from RAND Corp. and FCW.
from AI Trends https://ift.tt/3sqwy7X
via IFTTT
Comments
Post a Comment