Object Transplants Can Confound AI Machine Learning And Autonomous Cars
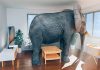
By Lance Eliot, the AI Trends Insider
Have you ever seen the videos that depict a scene in which there is some kind of activity going on such as people tossing a ball to each other and then a gorilla saunters through the background?
If you’ve not seen any such video, it either means you are perhaps watching too many cat videos or that you’ve not yet been introduced to the concept of inattentional blindness or what some consider an example of selective attention.
The gorilla is not a real gorilla, but instead a person in a gorilla suit (wanted to mention this in case you were worried that an actual gorilla was invading human gatherings and that the planet might be headed to the apes!).
Overall, the notion is that you become focused on the other activity depicted in the video and fail to notice that a gorilla has ambled into the scene.
Gorilla On The Mind
When I tell people about this phenomenon, and if they’ve not yet seen one of these videos, they are often quite doubtful that those viewing such videos really did not see the gorilla.
Of course, now that I’ve told you about it, you are not likely to be “fooled” by any such video since you have been forewarned about it.
Sorry about that.
Guess I should have said spoiler alert before I told you about the gorilla.
In any case, for those doubters about the assertion that people watching such a video are apt to not notice a gorilla (which, not noticing a squirrel might seem more plausible, I acknowledge), I assure you that a large number of cognition related experiments have been done with the “invisible gorilla” videos and such studies support this claim. The experiments tend to indicate that many people watching the video are completely unaware that a gorilla moseyed into the scene.
In fact, if you ask such people immediately after viewing the video whether they noticed a gorilla, most people swear it is outright impossible that a gorilla was in the scene.
Upon showing them the video a second time, they then see the gorilla, but also insist that you are tricking them by showing an identical video but one that you’ve sneakily inserted a gorilla into after-the-fact. These people will be utterly convinced that you are trying to trick them into falsely believing that the original video had a gorilla in it.
One experiment even involved videoing the person as they initially watched the gorilla related video, so that after being told about the gorilla, the person could watch the video of themselves watching the video, and hopefully then believe that there was a gorilla in the initial video.
I’m sure that there are some so suspicious that they figure you doctored the video of the video and thus refuse to still believe what they saw (or, what they didn’t see).
There is a bit of trickery somewhat involved in this matter.
Most of the time, the experimenter tells the person to be watching for something else in the video, such as watching to see if anyone drops the ball while tossing it or counting how many times the ball is tossed back-and-forth. I mention this rather important point because if you had no other focus related to the video, the odds are much higher that you would notice the gorilla. Because your attention has been purposely shaped though by the experimenter, you tend to block out other aspects that don’t pertain to the matter you were directed to pay attention to.
From a cognitive perspective, the aspect of not noticing the gorilla is at times attributed to inattentional blindness.
This means that you were not particularly paying attention to spot the gorilla and were therefore blind to noticing it. Others tend to describe this as an example of selective attention. Your attention is focused on something else that you were instructed to watch for. As a result, you select just those aspects in the scene that are related to the needed focus. No need to watch for other aspects and in fact it could be distracting if you did look elsewhere in the scene and therefore you might end-up doing worse on the assigned task at-hand.
The Elephant In The Room
Let’s switch now from talking about gorillas to instead talking about elephants.
You’ve likely heard the famous expression about there being an elephant in the room.
This is a popular metaphorical idiom and means that there is something that everyone notices or is aware of, but for which no one wants to bring it up or talk about it.
For example, I went to an evening party the other night and one of the attendees arrived wearing a quite unusual hat. The attendees were all exceedingly reserved and civil, and no one overtly pointed at the hat or made any direct explicit remarks about the hat. The hat was the elephant in the room. It was there but no one particularly spoke of it. We all saw it and knew that it was unusual.
This summer, I went to our local county fair and was able to stand in a room with an elephant. Yes, an actual elephant. In this case, everyone knew there was an elephant in the room and everyone spoke up about it and pointed at it. This was not a metaphorical elephant. Though, it certainly would have been more interesting if the elephant had been in the room and everyone pretended to not notice. I guess that might be somewhat dangerous though, if the elephant suddenly decided to lumber around in the room.
Anyway, some AI researchers recently conducted a fascinating study about an elephant in the room (of sorts).
It was a picture of an elephant.
The experiment they conducted dealt with the use of Machine Learning (ML) and Artificial Neural Networks (ANN).
Allow me to elaborate.
First, you might be aware that when using Machine Learning and deep neural networks that you need to contend with so-called adversarial examples.
This refers to the notion that some ML and ANN’s can be hyper-sensitive to targeted perturbations.
Let’s suppose you opt to train a neural network to be able to find images of turtles.
Little turtles, big turtles, turtles hiding in their shells, turtles poking their heads out, etc. You start by showing the neural network hundreds or maybe thousands of relatively crisp and clear-cut pictures of turtles. The neural network seems to gradually be getting pretty good at picking out the turtle in the pictures being shown to it. You test this by showing the neural network a picture that has a turtle and a squirrel, and sure enough the neural network is able to spot which of those animals is the turtle (and doesn’t misidentify the squirrel as being a turtle).
Great, you are ready to use the neural network.
But, suppose I do a bit of Photoshop work on the picture that contained the turtle and the squirrel. I make a copy of the squirrel’s tail and paste it onto the posterior of the turtle. I copy one of the legs of the turtle onto the squirrel. Admittedly, this looks somewhat Frankenstein-like, but go with me on this notion for the moment.
I show the picture to the neural network.
What could happen is that the neural network now reports that the turtle is not a turtle and asserts that there is not a turtle at all in the picture. Or, the neural network could assert that there are two turtles in the picture, falsely believing that the squirrel is also a turtle. How could this happen?
Sometimes, even a small perturbation can confuse the neural network.
If the neural network was focusing on only the legs of turtles, and it detected a turtle leg on the squirrel, it might then conclude that the squirrel is also a turtle. If the neural network had been honing on the tail of the turtle to determine whether a turtle is a turtle, the aspect that the turtle had a squirrel’s tail would have caused the neural network to no longer believe that the turtle is a turtle.
This is one of the known dangers, or let’s say inherent limitations, about the use of machine learning and neural networks.
A good AI developer will try to ascertain the sensitivity of the neural network to the various “factors” that the neural network had landed upon to do its detective work. Unfortunately, many deep neural networks are so complicated that it is not readily feasible to determine what it is using to do the detective work. All you have is a complicated set of mathematical aspects and for which you might not be able to “logically” discern what it refers to.
I’ve mentioned many times that this is something that AI self-driving cars need to be attuned to.
AI Autonomous Cars And Inattentive Attention
There are various studies that have shown how easy it can be to confuse a machine learning algorithm or neural network that might be used by an AI self-driving driverless autonomous car.
These are often used when the AI is examining the visual camera images being collected by the sensors of the self-driving car.
For several of my articles about notable machine learning and neural network limitations and concerns related to AI self-driving cars, see these:
https://aitrends.com/selfdrivingcars/ensemble-machine-learning-for-ai-self-driving-cars/
https://aitrends.com/ai-insider/machine-learning-benchmarks-and-ai-self-driving-cars/
https://aitrends.com/ai-insider/explanation-ai-machine-learning-for-ai-self-driving-cars/
https://aitrends.com/ai-insider/federated-machine-learning-for-ai-self-driving-cars/
At the Cybernetic AI Self-Driving Car Institute, we are developing AI software for self-driving cars. As such, we are actively using and developing these AI systems via the use of machine learning and neural networks. It’s important for the auto makers and tech firms to be using such tools carefully and wisely.
Allow me to elaborate.
First, I’d like to clarify and introduce the notion that there are varying levels of AI self-driving cars. The topmost level is considered Level 5. A Level 5 self-driving car is one that is being driven by the AI and there is no human driver involved. For the design of Level 5 self-driving cars, the automakers are even removing the gas pedal, the brake pedal, and steering wheel, since those are contraptions used by human drivers. The Level 5 self-driving car is not being driven by a human and nor is there an expectation that a human driver will be present in the self-driving car. It’s all on the shoulders of the AI to drive the car.
For self-driving cars less than a Level 5 and Level 4, there must be a human driver present in the car. The human driver is currently considered the responsible party for the acts of the car. The AI and the human driver are co-sharing the driving task. In spite of this co-sharing, the human is supposed to remain fully immersed into the driving task and be ready at all times to perform the driving task. I’ve repeatedly warned about the dangers of this co-sharing arrangement and predicted it will produce many untoward results.
For my overall framework about AI self-driving cars, see my article: https://aitrends.com/selfdrivingcars/framework-ai-self-driving-driverless-cars-big-picture/
For the levels of self-driving cars, see my article: https://aitrends.com/selfdrivingcars/richter-scale-levels-self-driving-cars/
For why AI Level 5 self-driving cars are like a moonshot, see my article: https://aitrends.com/selfdrivingcars/self-driving-car-mother-ai-projects-moonshot/
For the dangers of co-sharing the driving task, see my article: https://aitrends.com/selfdrivingcars/human-back-up-drivers-for-ai-self-driving-cars/
Let’s focus herein on the true self-driving car.
Much of the comments apply to the less than Level 5 and Level 4 self-driving cars too, but the fully autonomous AI self-driving car will receive the most attention in this discussion.
Here’s the usual steps involved in the AI driving task:
- Sensor data collection and interpretation
- Sensor fusion
- Virtual world model updating
- AI action planning
- Car controls command issuance
I’ve mentioned earlier in this discussion that one aspect to be careful about involves the potential of adversarial examples to confuse or mislead the AI system.
Object Transplants
There’s another somewhat similar potential difficulty involving what is sometimes called object transplants.
An interesting research study undertaken by researchers at York University and the University of Toronto provides an insightful analysis of the concerns related to object transplanting (the study was cleverly entitled “The Elephant in the Room”).
Object transplanting can be likened to my earlier comments about the gorilla in the video, though with a slightly different spin involved.
Imagine if you were watching the video that had a gorilla in it.
Suppose that you actually noticed the gorilla when it came into the scene.
If the scene consisted of people tossing a ball back-and-forth, would you be more likely to believe that it was a real gorilla or more likely to believe it is a fake gorilla (i.e., someone in a gorilla suit)?
Assuming that the people kept tossing the ball and did not get freaked out by the presence of the gorilla, I’d bet that you’d mentally quickly deduce that it must be a fake gorilla.
Your context of the video would remain pretty much the same as prior to the introduction of the gorilla.
The appearance of the gorilla did not substantially alter what you thought the scene consisted of.
Would the introduction of the gorilla cause you to suddenly believe that the people must be in the jungle someplace?
Probably not.
Would the gorilla cause you to start looking at other objects in the room and begin to think those might be gorilla related objects?
For example, suppose there was in the room a yellow colored stick.
Before the gorilla appeared, you noticed the stick and just assumed it was nothing more than a stick. Once the gorilla arrived, if you are now shifting mentally and thinking about gorillas, maybe the yellow stick now seems like it might be a banana. You know that gorillas like bananas. Therefore, something that has a somewhat appearance of a banana, might indeed be a banana.
I realize you might scoff at the idea that you would suddenly interpret a yellow stick to be a banana simply because of the gorilla being there. A child watching the video might be more susceptible to making that kind of mental leap. The child has perhaps not seen as many bananas in their lifetime as you have, and thus a yellow stick might seem visually close enough to the resemblance of a banana that a child would mistake it for such. The child didn’t think it was a banana before seeing the gorilla, it was the gorilla that caused the child to re-interpret the scene and the stick-like object in the context of a gorilla being there.
Visual object transplanting can impact the detection aspects of a trained machine learning system such as a convolutional deep neural network in a potentially similar way.
Using the popular Tensorflow object detection capability, and when combined with the Microsoft MS-COCO dataset, the researchers used a picture of a human sitting in a room and playing video games, and then did some object transplanting into the picture to see what the neural network would report about the objects in the picture (essentially, the researchers were doing a Photoshop-style transformation to the picture).
They transplanted an image of an elephant so that it appears in the picture with the sitting human.
In some instances, the neural network did not detect that the elephant was in the picture, presumably not even noticing that it was there (thus, the clever titling of the research study as dealing with the elephant in the room!).
Depending upon where the elephant was positioned in the picture, the neural network at one point reported that the elephant was actually a chair.
In another instance, the elephant was placed near other objects that had been earlier identified, such as cup and a book, and yet the neural network no longer reported having found the cup or the book. There were also instances of switched identifies, wherein the neural network had identified a chair and a couch, but with the elephant nearby to those areas of the picture, the neural network then reported that the chair was a couch and the couch was a chair.
You might complain about this experiment and say that it is perhaps “unfair” to suddenly place an elephant into a picture that has nothing to do with elephants.
The neural network had not been explicitly trained to have a co-occurrence of an elephant and the sitting human playing a video game. Well, the researchers considered this aspect and repeated the experiment but used a picture for which they merely took items already in the picture and moved those selected items around the scene. Once again, there were various inappropriate results produced by the neural network involving object misidentifications of one kind or another.
I would also suggest that we should decidedly not have much sympathy for the neural network per se and the aspect that it had not been trained on the co-occurrence possibilities – it’s inherent inability to readily cope with the co-occurrence aspects “on the fly” so to speak is a weakness that we must overcome overall for such AI systems.
AI Self-Driving Cars And Object Transplants
On a related note, I’ve previously mentioned in the realm of AI self-driving cars that there has been an ongoing debate related to the same notion of object transplanting, specifically the topic of a man on a pogo stick that suddenly appears in the street and near to an AI self-driving car.
For my article that discusses the pogo stick matter, see: https://aitrends.com/selfdrivingcars/egocentric-design-and-ai-self-driving-cars/
There are some AI developers that have argued that it’s understandable that the AI of a self-driving car might not recognize a man on a pogo stick that’s in the street.
By recognizing, I mean that the visual images captured by the self-driving car are examined by the AI and that the AI system was not able to discern that the object in the street consisted of a man on a pogo stick. It detected that an object was there, and had a rather irregular shape, but it was not able to discern that the shape consisted of a person and a pogo stick (in this instance, the two are combined, since the man was on a pogo stick and pogoing).
Why would it be useful or important to discern that the shape consists of a person on a pogo stick?
You, as a thinking human being, and assuming that you’ve seen a pogo stick before, and one that’s in use, you likely know that it involves going up-and-down and also moving forward or backward or side-to-side. If you were driving along and suddenly saw a person pogoing in the street, you’d likely be cognizant that you should be watching out for the potential erratic moves of the pogo stick and its occupant. You could potentially even predict which way the person was going to go, by watching their angle and how hard they were pogoing.
An AI system that merely construes the pogoing human as a blob would not readily be able to predict the behavior of the blob. Predictions are crucial when you drive a car. Human drivers are continually looking around the surroundings of the car and trying to predict what might happen next. That bike rider in the bike lane might be weaving just enough that you are able to predict that they will swerve into your lane, and so you take precautionary measures in-advance. We must expect AI self-driving cars, and especially the true Level 5 self-driving cars, must be able to do this same kind of predictive modeling.
The range of potential problems associated with object transplanting woes includes:
- In the case of object transplanting, there is the chance that the transplanted object is not detected at all, even though it might normally have been detected in some other context.
- Or, the confidence level or probability attached to the object certainty might be lessened in comparison to what it might otherwise have been (in the case of the elephant added into the picture and the subsequent missing cup or book, it could be that the neural network had detected the cup and the book but had assigned a very low probability to their identities, and so reported that they weren’t there, based on some threshold level required to be considered present in the picture).
- The detection of the transplanted object, if detection does occur, might lead to misidentification of other objects in the scene.
- Other objects might no longer be detected.
- Or, those other objects might have a lessened probability assigned to them as identifiable objects. There can be both local and non-local effects due to the transplanted object.
- Other objects might get switched in terms of their identities, due to the introduction of the transplanted object.
Conclusion
For AI self-driving cars, there are a myriad of sensors that collect data about the world surrounding the self-driving car. This includes cameras that capture pictures and video, it includes radar, it includes sonic, it includes LIDAR, and so on. The AI needs to examine the data and try to ferret out what the data indicates about the surrounding objects.
Are those cars ahead of the self-driving car or are they motorcycles?
Are there pedestrians standing at the curb or just a fire hydrant and a light post?
These are crucial determinations for the AI self-driving car and its ability to perform the driving task.
AI developers need to take into account the limitations and considerations that arise due to object transplanting. The AI systems of the self-driving car need to be shaped in a manner that they can sufficiently and safely deal with object transplantation and do so in real-time while the self-driving car is in motion. The scenery around the self-driving car will not always be pristine and devoid of unusual or seemingly out-of-context objects.
When I was a professor, each year a circus came to town and the circus animals arrived via train, which happened to get parked near the campus for the time period that the circus was in town. A big parade even occurred involving the circus performers marching the animals from next to the campus and over to the nearby convention center. It was quite an annual spectacle to observe.
I mention this because among the animals were elephants, along with giraffes and other “wild” animals. Believe it or not, on the morning of the annual parade, I would usually end-up driving my car right near to the various animals as I was navigating my way onto campus to teach classes for the day. It was as though I had been transported to another world.
If I was using an AI self-driving car, one wonders what the AI might have construed of the elephants and giraffes that were next to the car. Would the AI have suddenly changed context and assumed I was now driving in the jungle? Would it get confused and believe that the light poles were actually tall jungle trees?
I say this last aspect about the circus in some jest but do want to be serious about the facet that it is important to realize the existing limitations of various machine learning algorithms and artificial neural network techniques and tools. AI self-driving car makers need to be on their toes to prepare for and contend with object transplants.
And that’s no elephant joke.
That’s the elephant in the room and on the road ahead for AI self-driving cars.
Copyright 2020 Dr. Lance Eliot
This content is originally posted on AI Trends.
[Ed. Note: For reader’s interested in Dr. Eliot’s ongoing business analyses about the advent of self-driving cars, see his online Forbes column: https://forbes.com/sites/lanceeliot/]
from AI Trends https://ift.tt/2vllBw7
via IFTTT
Comments
Post a Comment