AI World Conference & Expo Hosts Its First AI Data Science Hackathon
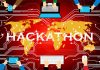
By Benjamin Ross
BOSTON—The AI World Conference & Expo featured its first ever AI Data Science Hackathon last week, which gave data scientists and developers from across the ecosystem the opportunity to solve real-world data challenges in applying artificial intelligence (AI) and machine learning.
Over the span of three days, teams worked to improve pipelines, datasets, tools, and other projects from a wide range of disciplines.
Two teams gave reports on their work to the AI World audience, one team focused on strategic planning powered by AI in the cloud, and the other working on a fractal AI model for versatility, speed, and efficiency.
Team one—designated “AI-Driven Strategy”—discussed the benefits of strategic planning for businesses with the assistance of AI. “I believe two things about strategic planning in most organizations,” the team’s leader said during their report out. “[First,] it has the potential to give an organization a powerful competitive advantage, if it’s performed competently. Second, it is usually not performed competently.”
AI-Driven Strategy’s solution is to apply AI and machine learning to automate the entire strategic planning function for every organization in the world. Of course, such an ambitious goal would take years to develop, the team leader says, and would reach a scale comparable to the Manhattan Project.
Ideally, the team’s strategy would include applying AI during the initial stage of strategic planning, which includes collecting data from four key domains: the resources and competencies within the organization; the targeted markets and customers; the industries and competitors potentially preventing the organization from reaching said markets and customers; and regulations, economics, demographics, and technologies that keep the organization on track.
For the Hackathon, the team attempted to develop algorithms that would take data dealing with markets and customers as they deal with healthcare organizations and create actionable insights.
“What we’re talking about here is a tool that’s going to bring strategic planning from the 19th century to the 21st century,” the team leader said. “This is a removal of the strategic planning model that would occur maybe once a year, where the top executives of a firm… would receive some data prepared by staff members and use their great wisdom acquired through ‘years of experience’ to make some decisions about strategic plans… Some attempt may have been made to implement them, but generally it wouldn’t work well, and as a result the organization would become disenchanted with the whole process.
“What we have in mind will be utterly dynamic, it’ll be happening all the time… Companies will be seeing these data constantly, and they’ll have the opportunity—with guidance from these algorithms—to make changes, to make adjustments, and to gain the competitive advantage that we believe is available through the strategic planning process.”
Hot Topic
Team two relied on an existing neural network architecture to tackle large spatial and time datasets. The architecture—called the Fractal Artificial Intelligence Model (FAIM)—was used by the team to predict the occurrence of forest fires in the U.S., with the end goal being to leverage that data to enable firefighters to take preventative action.
The team was led by FAIM’s co-founders, Jan Gerards and Jeroen Joukes, who told the audience an advantage of FAIM is its ability to analyze any kind of dataset quickly efficiently, and economically with a little amount of hardware needed. In fact, Gerards said, their work during the Hackathon was done entirely on a Raspberry Pi, a credit card-sized, low cost computer.
“We wanted to show the power of [FAIM], and the value propositions it can bring to the table,” said Gerards. “We hope that this can be disruptive in a positive way.”
The team looked at data from the Office of Satellite and Product Operations (OSPO), including longitude, latitude, temperature data, the size of a particular fire, and fire flags.
Joukes pointed out that FAIM works with previously uncollected data, calculating predictions in real time.
“Once the model is initiated, it trains on a historical dataset from scratch—which takes about five to ten seconds—and then it generates a bunch of predictions, stores them in a local database, and repeats,” Joukes said. “This cycle repeats over and over, collecting evidence for the future that you can use for all types of use cases where it is of the essence to make predictions quickly based on newly acquired information.”
While time constraints proved to be a major factor in the small portions of data collected by the team, Gerards reported that there are still lessons that can be learned from their work.
“I think the problems we faced makes this Hackathon—although we didn’t achieve our desired results— poignant because it’s a reminder that our machine learning capabilities and the abilities to enable AI to provide solutions is really constricted by our data,” said Gerards. “[Data] needs to be cleaned, it needs to be properly packaged together. Otherwise the tool is useless.”
from AI Trends https://ift.tt/36rQZHF
via IFTTT
Comments
Post a Comment