Tesla’s 500,000 Vehicles on the Road Give it a Lead in Generating Data for its Self-Learning AI Self-Driving Cars
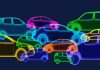
By AI Trends Staff
Tesla is making its own way in the application of AI to self-driving cars, making several key decisions that contrast with designs of other AI self-driving car software developers, and having the advantage of a large installed base of vehicles on the road.
One early decision was to make over the air (OTA) software updates available to Tesla drivers. In October 2015, each of Tesla’s 60,000 owners received an OTA update, based on data gathered for a year from all Tesla drivers. In 2014, Tesla sent a software fix for overheating to its 30,000 owners at the time, according to an account in TEchiexpert.
Tesla has compiled data from over 100 million miles driven with its autopilot software. The is being used to generate roadmaps for self-driving cars, which Tesla claims are 100 times more accurate than alternative navigation systems. The company is considering whether to offer the data for sale to other automakers, or possibly offer it to the government to help make roads safer.
McKinsey and Co. has estimated the market value for vehicle-gathered data will be some $750 billion a year by the end of 2030.
Today Tesla is estimated to have 500,000 vehicles on the road, driving 15 million miles a day, which is 5.4 billion miles per year. That is more than 200 times the expected experience from Waymo a year from now, according to an account in Towards Data Science. And Tesla is adding 5,000 cars per week to its fleet.
In the areas of computer vision, prediction and path planning, this data makes a difference. Object detection will become better over time, especially for rare instances such as a horse in the road. People are still being paid to manually label or tag images, a bottleneck. But Tesla is in a position to take snapshots, contributing images from driving experience. Tesla’s director of AI, Andrej Karpathy, has established a process for sourcing images to train for object detection.
Anthony Levandowski, a former top engineer at Waymo, has said full autonomy for self-driving cars will be difficult to achieve, in part because “today’s software is not good enough to predict the future,” and a primary category of failure is to predict the behavior of nearly cars and pedestrians.
Tesla has a self-learning advantage from its fleet of 500,000 vehicles on the road. Instead of having humans label images, Tesla’s prediction neural network can learn correlations between the past and future from sequences of events. They can see behavior that preceded behavior in any recording.
Path planning involves staying in the lane, changing lanes, changing speeds, going around parked cars, stopping for jaywalkers, and so on. This is difficult to code. One approach is to get a neural network to copy the actions of human drivers, in what is known as imitation learning, or apprenticeship learning. The neural network draws correlations between what it sees via computer vision, and the actions taken by human drivers. Tesla will be extending imitation learning to more tasks over time, such as changing lanes. Tesla can target “replays” of situations it wants to study, such as left turns.
Tesla appears to have the lead in generating training data that feeds its self-learning systems, a lead it will be difficult to close for other self-driving car automakers.
Read the source articles in TEchiexpert and Towards Data Science.
from AI Trends https://ift.tt/2Hy2lyH
via IFTTT
Comments
Post a Comment